Neste tutorial, veremos como realizar o reconhecimento de objetos com o Yolo e o OpenCV usando uma rede neural pré-treinada usando o aprendizado profundo.
Vimos num tutorial anterior como reconhecer formas simples utilizando a visão por computador. Este método só funciona para certas formas simples predefinidas. Se quiser reconhecer uma maior variedade de objectos, a forma mais fácil é utilizar a inteligência artificial.
Hardware
- Um computador com uma instalação Python3
- Uma câmara
Princípio
A inteligência artificial é um domínio da informática em que o próprio programa aprende a realizar determinadas tarefas. O reconhecimento visual, em particular. Neste tutorial, vamos utilizar uma rede neural treinada para reconhecer formas específicas.
São necessários muitos dados para poder treinar corretamente uma rede neural. Foi demonstrado que a aprendizagem é mais rápida numa rede neuronal treinada para outra coisa. Por exemplo, uma rede neuronal treinada para reconhecer cães será treinada mais facilmente para reconhecer gatos.
A Ultralytics oferece os modelos YOLO, que são fáceis de utilizar e bastante eficazes na deteção, classificação e monitorização.
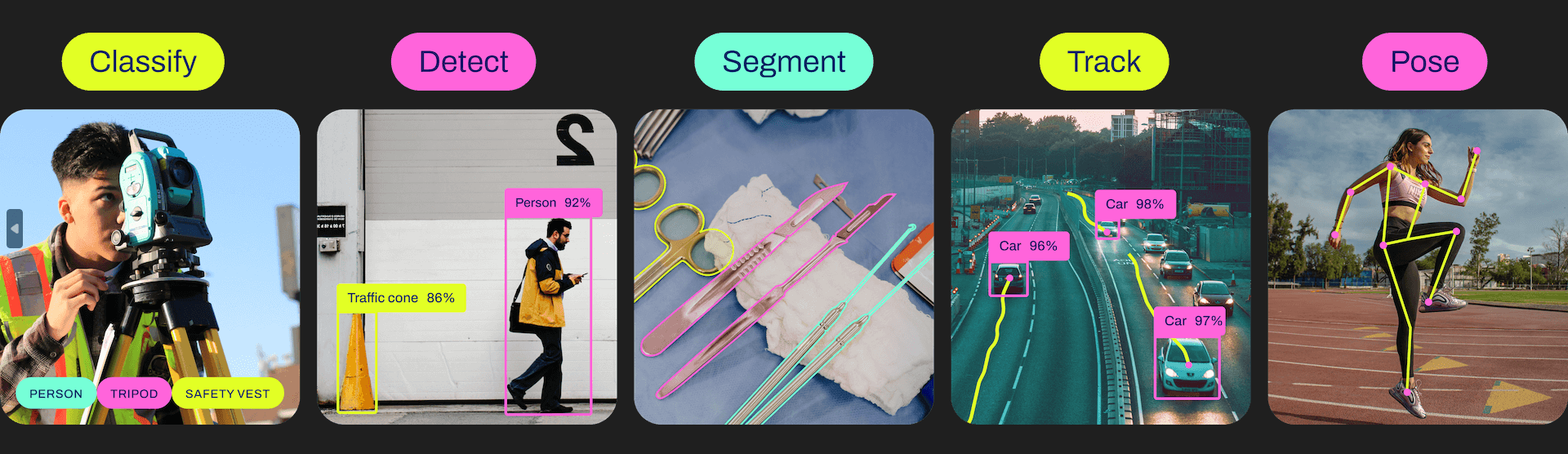
Para cada versão, existem diferentes modelos, desde o mais leve e menos potente até ao mais potente mas mais pesado.
YOLOv5n | YOLOv5s | YOLOv5m | YOLOv5l | YOLOv5x |
YOLOv8n | YOLOv8s | YOLOv8m | YOLOv8l | YOLOv8x |
Configurar o Python
Caso contrário, pode descarregar e instalar o Python 3
Pode então instalar as bibliotecas necessárias: imutils, OpenCV, ultralytics, etc.
python3 -m pip install imutils opencv-python ultralytics
Recuperar um modelo pré-treinado
É possível obter um modelo pré-treinado a partir do script pyhton
# load the pre-trained YOLOv8n model model = YOLO("yolov8n.pt")
Script Python para reconhecimento de objectos
Em primeiro lugar, criamos um fluxo de vídeo (vs) utilizando a biblioteca imutils, que irá obter as imagens da câmara.
vs = VideoStream(src=0, resolution=(1600, 1200)).start()
Inicializamos uma rede neural com os parâmetros do modelo utilizando a biblioteca YOLO (yolov5, v8, etc.).
model = YOLO("yolov8n.pt")
Em seguida, criaremos um ciclo que, em cada iteração, lerá a imagem da câmara e passá-la-á para a entrada da rede neural para deteção e reconhecimento de objectos.
while True: # start time to compute the fps start = datetime.datetime.now() #ret, frame = video_cap.read() #frame = vs.read(); ret=True # if there are no more frames to process, break out of the loop if not ret: break # run the YOLO model on the frame detections = model(frame)[0]
Por fim, o código apresenta a caixa de deteção na imagem, a probabilidade de reconhecimento e a posição.
# loop over the detections for box in detections.boxes: #extract the label name label=model.names.get(box.cls.item()) # extract the confidence (i.e., probability) associated with the detection data=box.data.tolist()[0] confidence = data[4] # filter out weak detections if float(confidence) < CONFIDENCE_THRESHOLD: continue # if the confidence is greater than the minimum confidence xmin, ymin, xmax, ymax = int(data[0]), int(data[1]), int(data[2]), int(data[3]) cv2.rectangle(frame, (xmin, ymin) , (xmax, ymax), GREEN, 2) #draw confidence and label y = ymin - 15 if ymin - 15 > 15 else ymin + 15 cv2.putText(frame, "{} {:.1f}%".format(label,float(confidence*100)), (xmin, y), cv2.FONT_HERSHEY_SIMPLEX, 0.5, GREEN, 2)
Código completo de reconhecimento de objectos com OpenCV e YOLO
#!/usr/bin/env python # -*- coding: utf-8 -*- # import datetime from ultralytics import YOLO import cv2 from imutils.video import VideoStream #from helper import create_video_writer # define some constants CONFIDENCE_THRESHOLD = 0.8 GREEN = (0, 255, 0) # load the pre-trained YOLOv8n model model = YOLO("yolov8n.pt") #model = YOLO("yolov5n.pt") """ #detect on image frame= cv2.imread('./datasets/two-boats.jpg');ret= True #from image file detections = model(frame)[0] # loop over the detections #for data in detections.boxes.data.tolist(): for box in detections.boxes: #extract the label name label=model.names.get(box.cls.item()) # extract the confidence (i.e., probability) associated with the detection data=box.data.tolist()[0] confidence = data[4] # filter out weak detections by ensuring the # confidence is greater than the minimum confidence if float(confidence) < CONFIDENCE_THRESHOLD: continue # if the confidence is greater than the minimum confidence, # draw the bounding box on the frame xmin, ymin, xmax, ymax = int(data[0]), int(data[1]), int(data[2]), int(data[3]) cv2.rectangle(frame, (xmin, ymin) , (xmax, ymax), GREEN, 2) #draw confidence and label y = ymin - 15 if ymin - 15 > 15 else ymin + 15 cv2.putText(frame, "{} {:.1f}%".format(label,float(confidence*100)), (xmin, y), cv2.FONT_HERSHEY_SIMPLEX, 0.5, GREEN, 2) # show the frame to our screen cv2.imshow("Img", frame) """ #detect on video # initialize the video capture object #vs = VideoStream(src=0, resolution=(1600, 1200)).start() video_cap = cv2.VideoCapture("datasets\\Splash - 23011.mp4") # initialize the video writer object #writer = create_video_writer(video_cap, "output.mp4") while True: # start time to compute the fps start = datetime.datetime.now() #ret, frame = video_cap.read() #frame = vs.read(); ret=True # if there are no more frames to process, break out of the loop if not ret: break # run the YOLO model on the frame detections = model(frame)[0] # loop over the detections #for data in detections.boxes.data.tolist(): for box in detections.boxes: #extract the label name label=model.names.get(box.cls.item()) # extract the confidence (i.e., probability) associated with the detection data=box.data.tolist()[0] confidence = data[4] # filter out weak detections by ensuring the # confidence is greater than the minimum confidence if float(confidence) < CONFIDENCE_THRESHOLD: continue # if the confidence is greater than the minimum confidence, # draw the bounding box on the frame xmin, ymin, xmax, ymax = int(data[0]), int(data[1]), int(data[2]), int(data[3]) cv2.rectangle(frame, (xmin, ymin) , (xmax, ymax), GREEN, 2) #draw confidence and label y = ymin - 15 if ymin - 15 > 15 else ymin + 15 cv2.putText(frame, "{} {:.1f}%".format(label,float(confidence*100)), (xmin, y), cv2.FONT_HERSHEY_SIMPLEX, 0.5, GREEN, 2) #cv2.circle(frame, (int(X)-15, int(Y)), 1, GREEN, 2) #cv2.putText(frame, poslbl, (int(X), int(Y)),cv2.FONT_HERSHEY_SIMPLEX, 0.5, GREEN, 2) # end time to compute the fps end = datetime.datetime.now() # show the time it took to process 1 frame total = (end - start).total_seconds() print(f"Time to process 1 frame: {total * 1000:.0f} milliseconds") # calculate the frame per second and draw it on the frame fps = f"FPS: {1 / total:.2f}" cv2.putText(frame, fps, (50, 50), cv2.FONT_HERSHEY_SIMPLEX, 2, (0, 0, 255), 4) # show the frame to our screen cv2.imshow("Frame", frame) #writer.write(frame) if cv2.waitKey(1) == ord("q"): break video_cap.release() vs.stop() #writer.release() cv2.destroyAllWindows()
Fontes de imagem para deteção de objectos
Pode utilizar este script com diferentes fontes de imagem. Para tal, é necessário adaptar ligeiramente o código anterior para modificar a variável “img” que contém a imagem a analisar.
- A câmara Web do seu computador
vs = VideoStream(src=0, resolution=(1600, 1200)).start() while True: frame = vs.read()
O fluxo de vídeo deve ser interrompido no final do guião com vs.stop()
- A câmara IP
vc = cv2.VideoCapture('rtsp://user:password@ipaddress:rtspPort') while True: ret, frame=vc.read() #from ip cam
Certifique-se de que pára a captura de vídeo no final do guião com vc.release()
- O Raspberry Pi Picam
vs = VideoStream(usePiCamera=True, resolution=(1600, 1200)).start() while True: frame = vs.read()
Lembre-se de parar o fluxo no final do script com vs.stop()
- Um ficheiro de vídeo
vc = cv2.VideoCapture('./datasets/Splash - 23011.mp4') #from video while True: ret, frame=vc.read() #from video
- Um ficheiro de imagem
frame= cv2.imread('./datasets/two-boats.jpg')
Resultados
Para este exemplo, enviamos uma imagem de dois barcos como entrada para a rede neural, que são corretamente reconhecidos. Para obter resultados ligeiramente diferentes, pode modificar o parâmetro de confiança para evitar falsos positivos.
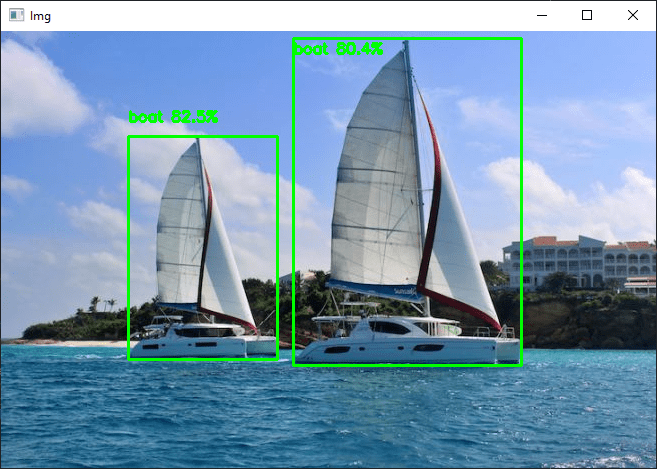
Pode testar este código com a sua webcam ou com fotografias, por exemplo, para ver o desempenho do modelo e do reconhecimento de objectos.
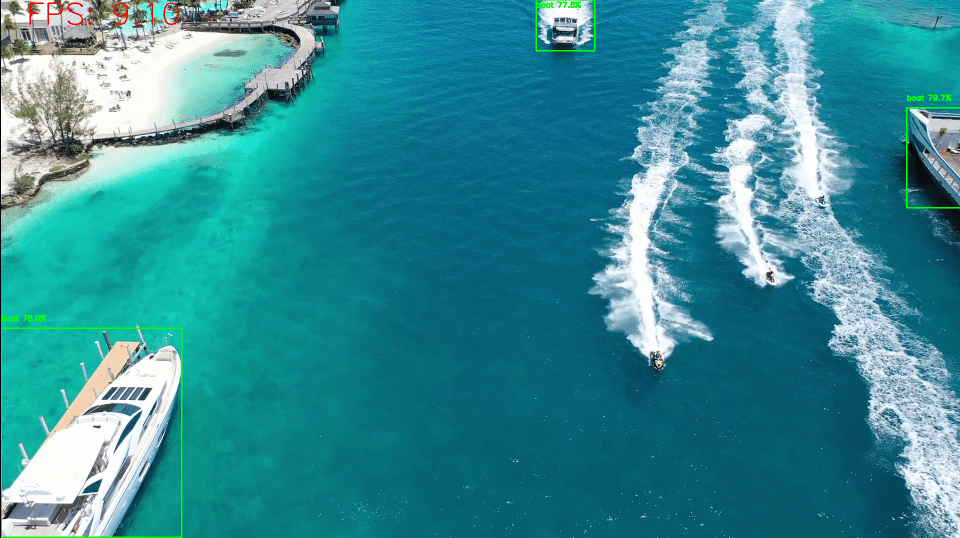
Quando o script estiver a funcionar, pode treinar o modelo para detetar outros objectos.